Sign up for the DPO Europe Newsletter
We will share useful materials with you and talk about the latest news from the world of privacy.
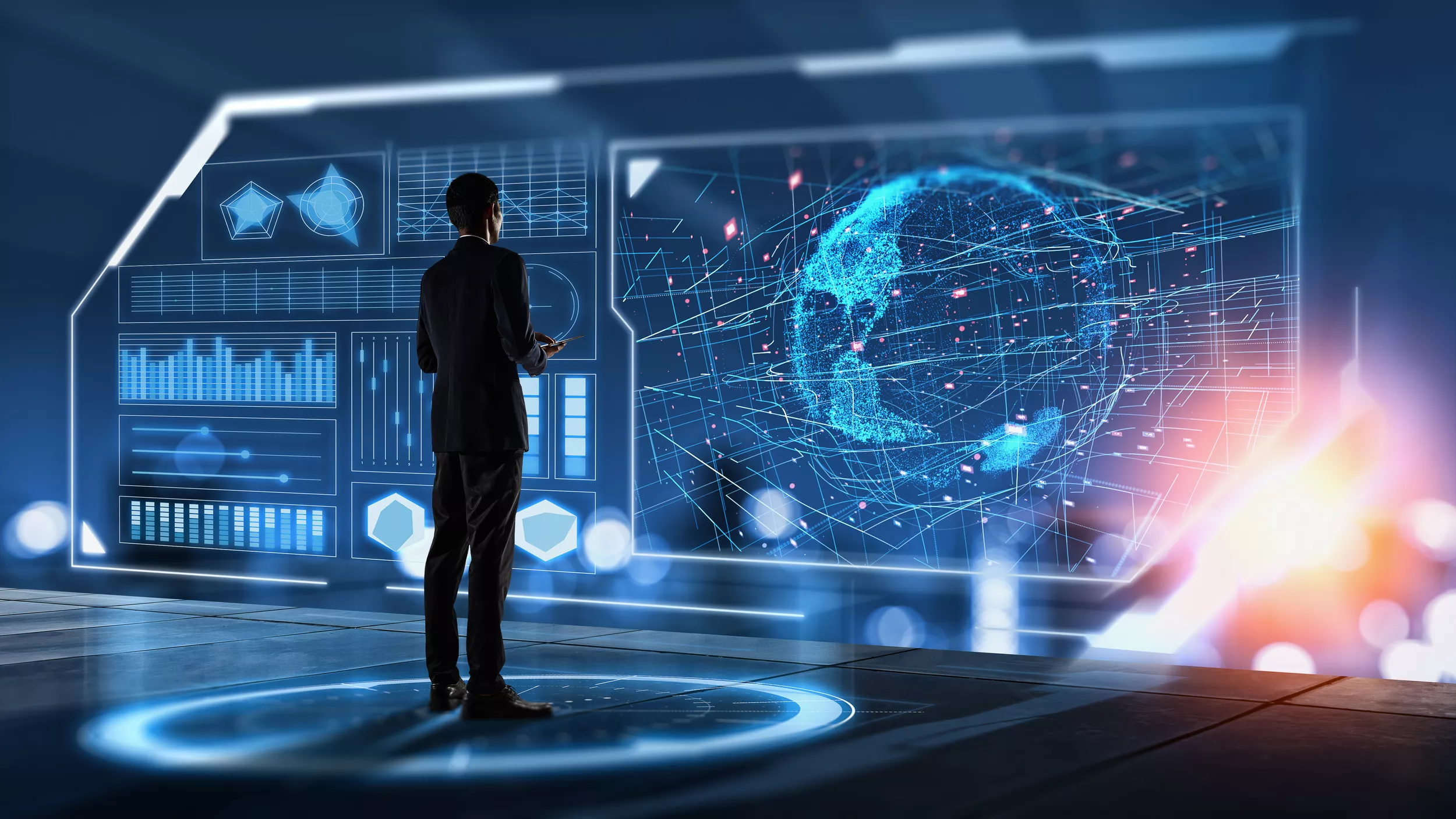
Guide to Artificial Intelligence: How Companies Can Keep Users’ Privacy in Mind
- AI, Artificial Intelligence, Privacy
- 04/03/2024
- Author: Elena Aliseychik, Junior Consultant
In this article, we will look at the different types of AI models, their inherent privacy risks, and promising solutions that can help protecting users’ data. It is important to highlight that adopting strategies to limit or ban the use of AI is become a lesser realistic solution for businesses. Instead, the focus should be on finding innovative approaches and technologies that can coexist with AI and minimize potential threats to privacy.
The Importance of Privacy in the AI Era
Artificial Intelligence (AI) has become a transformative force that infiltrates various aspects of society and changes the way we live, work and interact. AI technologies have recently experienced unprecedented growth, driving innovation across all industries and providing solutions to previously complex challenges. In the healthcare industry, AI drives breakthroughs in diagnosis, medicine discovery and personalized treatment plans. In finance, algorithms analyse vast amounts of data to optimize investment strategies and detect fraudulent activity. Educational platforms are using AI to tailor the learning process to individual student needs. The entertainment industry is using AI to personalize content and increase user engagement.
The proliferation of smart devices and the shift to the Internet of Things (IoT) amplifies AI’s impact on our lives, creating a digital ecosystem where intelligent systems are constantly learning, adapting and evolving with minimum need for direct user involvement.
As society increasingly relies on AI-driven solutions, the balance between technological advancement and privacy becomes a necessity. Nowadays, when AI systems can understand, predict, and influence human behaviour, protecting personal data becomes the fundamental aspect of preserving the right to make choices without external coercion. Privacy ensures that people retain control over their personal data, and thus over the consequences of processing it.
Types of AI Models and Their Implications on Privacy
Supervised Learning
Supervised learning is a machine learning paradigm in which an algorithm is trained on a set of pre-labelled data consisting of input-output pairs. For example, if an algorithm is learning to recognize pictures of cats, it will be shown a set of cat pictures with the label “cat” during training. The model itself recognizes which features correspond to what is labelled “cat”. When a new photo arrives as the input that AI hasn’t seen before, it uses its experience to tell whether it looks like a cat or not. This approach is applicable in many tasks: recognizing images, converting speech to text, or identifying spam in emails.
Although supervised learning has proven to be highly effective in various domains, the process of processing labelled datasets raises serious privacy concerns.
Information Disclosure
Supervised learning is a machine learning paradigm in which an algorithm is trained on a set of pre-labelled data consisting of input-output pairs. For example, if an algorithm is learning to recognize pictures of cats, it will be shown a set of cat pictures with the label “cat” during training. The model itself recognizes which features correspond to what is labelled “cat”. When a new photo arrives as the input that AI hasn’t seen before, it uses its experience to tell whether it looks like a cat or not. This approach is applicable in many tasks: recognizing images, converting speech to text, or identifying spam in emails.
Although supervised learning has proven to be highly effective in various domains, the process of processing labelled datasets raises serious privacy concerns.
The Difficulty of Securing Informed Consent
Obtaining consent to include personal data in training sets is crucial. Consent should be informed, but sometimes users don’t think about the consequences of having their data processed. This is because AI technologies and processes can be challenging for non-specialists to understand. Data subjects are not always given sufficient information about exactly how their data will be used. That means they can’t make an informed decision about the consent to data processing.
Daniel Solow in his recent , Daniel Solow looks at various examples of companies that specify that data can be used to train artificial intelligence. He emphasizes that these formulations may either go unnoticed by users or only superficially perceived due to the complexity of the technical aspects. Solow also notes that even consent collected according to all formal rules does not always provide data subjects with comprehensive insights, especially in the context of artificial intelligence use. Developers need to make sure that people know how their data will be used and give them the opportunity to opt out of this processing. Article reviews various examples of companies that specify that data can be used to train artificial intelligence. He emphasizes that these formulations may either go unnoticed by users or only superficially perceived due to the complexity of the technical aspects. Solow notes that even consent collected according to all formal rules does not always provide data subjects with comprehensive insights, especially in the context of AI use. Developers need to make sure that people know how their data will be used and give them the opportunity to opt out of this processing.
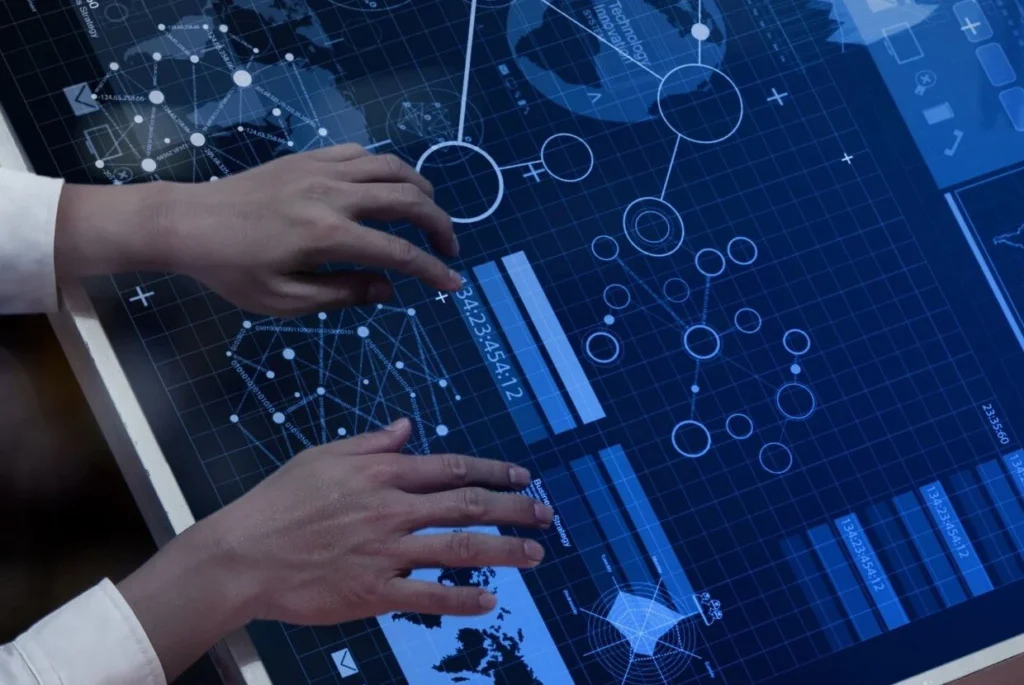
Data Aggregation and Re-identification
Aggregation of labelled data from multiple sources can inadvertently lead to re-identification of people. Even if specific identifiers are removed, the combination of different datasets can sometimes restore a person’s identity or distinguish them from others. For example, the article “Re-Identification of ‘Anonymized Data” cites an example where an intern at Neustar was able to use a cab medallion number, trip time and date to link a dataset of trips to images of celebrities. As a result, he was able to re-identify some of the trip data and obtain additional personal information.
Bias and Discrimination Issues
The unbiasedness of AI algorithms is directly dependent on the data on which they are trained. If the training set is not properly formed, the data can be used to perpetuate existing biases or inadvertently introduce new ones.
Developers must be careful to avoid training discriminatory models and ensure that the training data is representative of different demographic groups to minimize the risk of unintentional discrimination. Technical solutions to this problem already exist. For example, Faisal Kamiran and Toon Calders have proposed a machine learning method that addresses the bias problem. The idea is to make small changes to the training data so that it becomes more balanced. In this way, the differences between data about different groups of subjects are first smoothed out, and then a model is trained on this modified data that will not exhibit bias but will maintain the necessary predictive accuracy.
Data Security and Storage
The labelled training datasets must also be securely stored to prevent unauthorized access or data leakage. Solving the data security problem in supervised learning involves the delicate balance between leveraging the power of labelled datasets for effective model training and protecting the personal information that is in those datasets. As AI technologies evolve, addressing these issues becomes paramount to building trust and ensuring responsible AI system design.
Unsupervised Learning
Unsupervised Learning is a type of machine learning in which algorithms analyse and identify patterns in datasets without explicit instructions or labelled results. The main goal is to identify inherent structures or relationships in the data. Let’s imagine that you have a set of photos, and you want the system to find which objects are similar. Instead of specifying that one photo has a cat, and another has a dog, developer gives the system the ability to look for common features or groups that may unite the photos on its own. Examples of using teacherless learning are clustering, dimensionality reduction, and anomaly detection.
While teacherless learning, can be an effective and popular way to extract meaningful inferences from data, it poses unique privacy concerns, especially while analysing and clustering data.
Unintentional Recognition
This problem is closely related to the concept of overfitting. If the model does not have enough data to recognize general patterns, it may retain some of the training data without drawing conclusions from it. On the other hand, too much data can also be a danger if the model is too complex and adjusts for every detail in the data instead of identifying general patterns. In this way, the model seems to “remember” the data it was trained on rather than in generalized values.
So, what is the danger of overfitting for privacy? The point is that in the end a neural network is a file that is transferred from the developer to the customer, and from the customer to other users. Along with the neural network, the data that has been “memorized” will also be transferred.
In this way, the article “Privacy Risk in Machine Learning: Analyzing the Connection to Overfitting” is quite interesting. The authors proved that “overfitting” of popular AI algorithms leads to the vulnerability of data training. The resulting model remains stable enough to continue to be used, but at the same time it reveals precise information on whether an individual’s data belongs to the training set or not.
Limited Discovery Control
In teacherless learning, the algorithm independently discovers patterns without explicit human control. This lack of control creates issues in preventing the AI from making associations that violate privacy or inferences that could harm humans. This underscores the importance of closely monitoring the performance of teacherless algorithms and introducing safeguards to prevent such problems.
For example, a version of the European Union’s Artificial Intelligence Regulation that has recently hit the web proposes the increased oversight of “high-risk” systems. They would include models that process medical data or are used by HR departments.
Reinforcement Learning
Let’s imagine, we have an artificial intelligence that is eager to learn how to make right decisions in some situations by interacting with the environment. When the AI acts, the environment corresponds whether the action was good (and brings a reward) or bad (imposes a punishment). The AI seeks to improve its actions to maximize the total reward over time.
The classic example of reinforcement learning is AlphaGo, which learns to play the game of Go by receiving rewards for winning. This also includes autonomous systems, such as robot control algorithms that can learn to perform tasks in the real world based on feedback from their actions. Such a “trial and error” process can raise special privacy concerns.
Learning from Real-World Interactions
Reinforcement learning often involves interactions with the real-world environment, which may contain information about the individual. The system learns from these interactions, and if not properly supervised, it may inadvertently adapt to private details, posing a privacy risk.
Let’s suppose that we are training an autonomous robot in the environment that interacts with furniture, sensors, and, perhaps, even people. If the system is trained without due diligence, there is a risk that it may accidentally learn and memorize some unique details (e.g. the location of personal items in the home).
Previously, we have discussed the risk of “memorizing” training data (see the overfitting), but reinforcement learning is dangerous because such systems often have contact directly with a person’s “habitat”, their home or digital space.
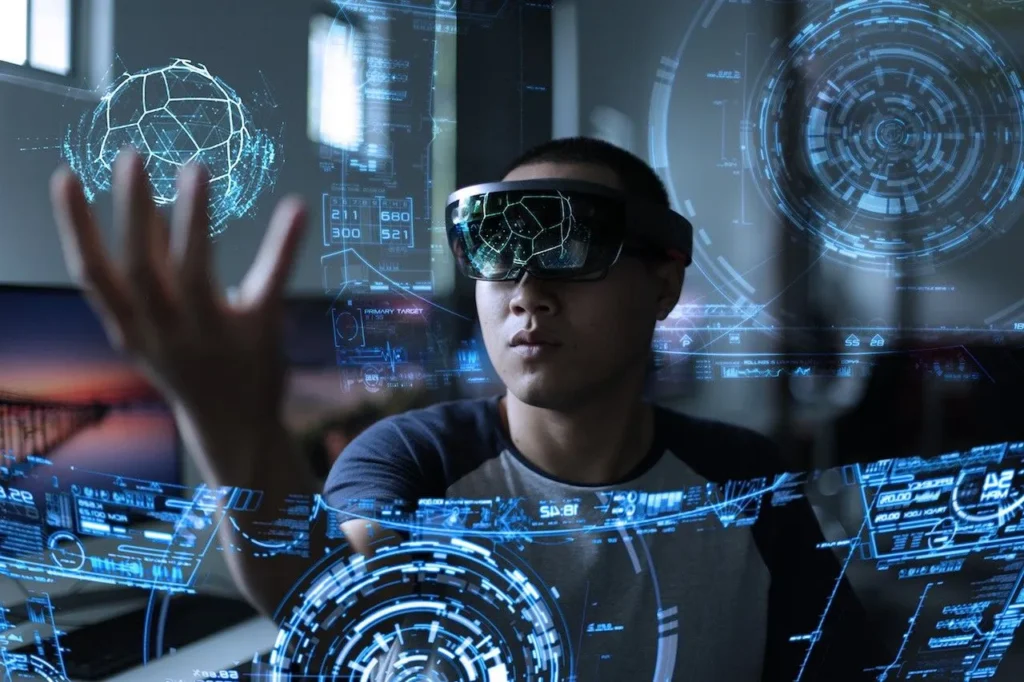
Risks of Transfer
The problem with transferring reinforcement learning to another environment is that models trained in one environment may be applied in another one where different privacy rules are applied. This can create a risk of misapplication of learned strategies and have unintended consequences for data protection (for example, if we train a model in an environment with lenient privacy rules and then apply it in another environment with more stringent requirements).
A model that is built in one environment may not consider the features or limitations of another, leading to undesirable consequences. This risk is discussed in the article “Robust Adversarial Reinforcement Learning with Dissipation Inequality Constraint”. The authors took into the consideration the differences between training and real-world environments as a type of security attack and propose a method that makes the system more resilient to change.
Nevertheless, it is important to consider the context and privacy rules in all phases of training reinforcement models. Also, it’s vital to be careful when applying them in new environments. This will help to avoid potential risks and comply with privacy regulations in different contexts.
Risks Associated with Human Involvement
When humans become part of the reinforcement learning cycle, there is a significant risk that the system may learn based on their actions and preferences. Ensuring respect for user privacy and avoiding the usage of sensitive information become critical to the ethical deployment of such systems. For example, if we use reinforcement learning to create personalized recommendations or assistants that interact with users, it is important that these systems do not store or use personal data without consent. This could be personal preferences, medical information, or other sensitive aspects of a user’s life.
To ensure that reinforcement learning respects privacy standards, proper control over what data is used in training scenarios and how that it is stored and processed becomes necessary. Additionally, implementing privacy mechanisms such as data anonymization or access control is an important step to protect sensitive user information.
Generative Models
Generative models are the type of machine learning algorithms aiming to reproduce the underlying patterns of a training dataset. These models generate new data instances similar to the original data. Generative models find applications in image synthesis, text generation, and data augmentation.
In addition to the danger of “overlearning” and reproduction of data from training sets, which is also relevant for this type of algorithms: deepfakes and misinformation are the most common privacy concerns.
Generative models, especially GANs, can be used to create fake content that looks like realistic images and videos. Synthetic data is used to misinform, steal identities, or create misleading narratives that can affect people’s reputations and privacy. In politics, for example, deepfakes are used to create deceptive videos, such as the 2018 deepfake with former US President Barack Obama discussing fake news, or the video of Nancy Pelosi that went viral in 2019, where her speech was deliberately slowed down to give the impression of intoxication. Deepfakes can also be used for phishing purposes, creating bogus data that misleads users and can be used for privacy attacks.
Opportunities to Strengthen the Personal Data Protection
Let’s briefly review techniques to improve data security in the context of machine learning. We will focus on two important data security techniques: federated learning and differentiated privacy.
Federated learning is the way to train AI models without centralizing data. Instead of collecting all the data in one place, models are given the ability to be trained directly on devices. For example, federated learning allows your smartphone to participate in training a model on its own without sending your personal information to a central server. This means that a user can receive personalized recommendations or services, but only their device will have access to the data and analysis results.
Differential privacy is the way of processing data in a way that safeguards its confidentiality. When a developer is going to extract useful information from a group of data, they add a little random “noise” to each piece. Therefore, even if someone recognizes their own data in this overall analysis, they won’t be able to determine exactly what information belongs to other people. For example, employee salary data is processed by the system. Before training the model, the developers add a small amount of random noise to each amount. At the same time, the noise level is calculated mathematically, and the results of data analysis are not distorted. Thus, the algorithm can calculate the overall average salary without revealing the exact salaries of each employee. This approach is used to make data analysis useful without violating people’s privacy. It is often used in medical research, employment statistics, and other areas where it is important to maintain privacy when processing information.
Potential Applications of AI in Enhancing Privacy
Artificial intelligence should not be viewed solely as a source of potential risks; on the contrary, it gives new opportunities for privacy. Automated audits, analysing policies for language accessibility – all these methods can effectively detect and prevent privacy violations.
Artificial intelligence also facilitates predictive analytics, allowing you to predict potential risks and take preventive action. Automated incident response, data discovery and classification, AI-powered encryption and tokenization tools strengthen data security and facilitate compliance.
Dashboards, risk analytics, and machine learning-based risk assessment integrated into privacy management processes provide a comprehensive approach to data protection. These technologies not only increase efficiency but support proactiveprivacy management to help organizations keep pace with new regulatory requirements.
Conclusion
In the era of AI, privacy is becoming not only a legal imperative, but an ethical obligation for developers, organizations, and policymakers. Finding the balance between technological innovation and privacy protection is vital to building a future where technology enhances our well-being.
AI is not an inevitable enemy, but rather a powerful tool that can not only provide effective data management, but also help protect data. Various AI models carry their own specific privacy risks, but this only emphasizes the need to use them ina a wise and responsible way.
Understanding and managing the risks associated with the use of AI are becoming the key aspect of successfully integrating this technology. It is important to consider not only the potential threats, but also the opportunities AI presents to protect privacy. The balanced approach to AI implementation that combines effective auditing techniques, automated incident response systems, risk analysis, and encryption technologies will boost the benefits of AI and minimize privacy risks.
The further expansion of the AI systems depends on public confidence in the technology. People are more likely to embrace technological innovation if they are sure that their personal information is treated with care and respect for their privacy. Ensuring robust privacy protections helps build trust in artificial intelligence systems and foster a positive relationship between technology and society.
Contact us
Fill out the form and we will contact you as soon as possible!
Our team’s expertise and their qualifications enable us to tackle any challenge related to the implementation of personal data protection and other privacy-related issues.