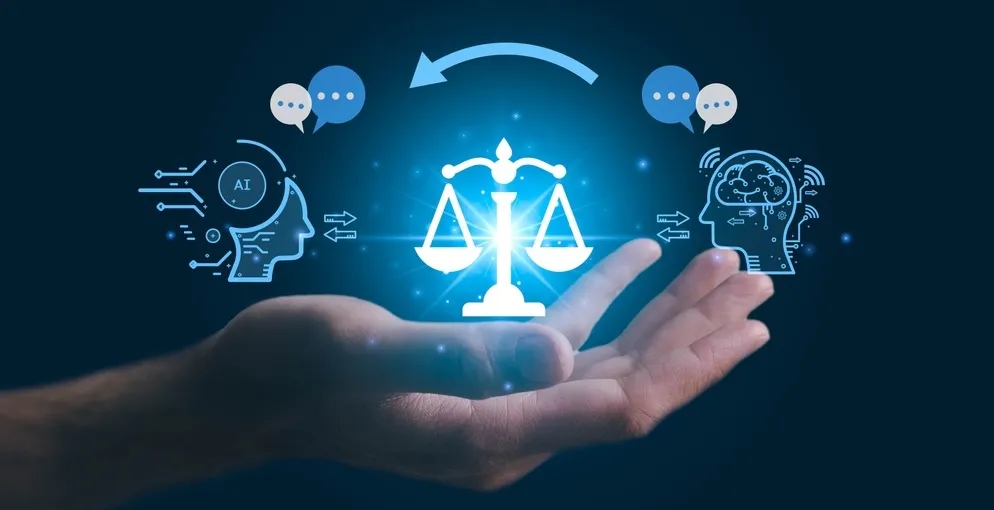
10 important questions lawyers should ask technical teams about AI systems
- AI, Artificial Intelligence, Privacy
- 06/12/2024
To properly assess whether an AI system brings any privacy risks, you need to ask your technical teams the right questions.
AI and privacy: 10 questions data privacy officer to ask technical team about AI systems
With the rise of AI and the advent of the European Union’s AI Regulation (EU AI Act), lawyers are playing a key role in ensuring that companies comply with the new rules. This law introduces common standards for the management of AI systems and focuses on systems that can have a significant impact on people’s lives and rights. Many of the issues are based on articles that relate to high-risk systems. However, it is important to remember that even AI systems that do not fall into this category can pose potential threats. Understanding how they work, how they process data and what privacy risks may arise is an integral part of an overall strategy to protect user rights and comply with the law.
To properly assess whether an AI system is safe and compliant with the EU Regulation, you need to ask your technical teams the right questions.
In this article, we look at 10 important questions lawyers can ask technical teams.
What is the purpose of the AI system and in what situations will it be used?
Understanding exactly what the purpose of the system is and how it will be used is essential. It helps to understand what legal requirements need to be applied.
Firstly, it helps to determine if the system is high risk. Secondly, knowing the purpose of the system is necessary to assess whether it meets the legal requirements for accuracy, reliability and security. Third, it adds transparency in understanding the capabilities and limitations of AI.
Examples of answers to this question might include: the system is designed to analyse medical images and detect cancerous tumours; it is used to optimise logistics in large warehouses; the system analyses financial data to detect fraud. If AI is used in important areas such as healthcare or justice, it automatically indicates high risks and stricter legal requirements.
How does the AI system ensure the quality and relevance of the personal data that is used to power it?
Data quality and relevance are of utmost importance: errors or flaws in the data can lead to inaccurate results or bias.
Why is it important to ask this question?
📎 Compliance with the law: in particular, Article 10 of the Regulation requires that clear data quality assurance procedures are established for high-risk systems.
📎 Even if the system is not high risk, the accuracy and reliability of the AI depends on the data being of high quality and representative.
📎 The right approach to data helps avoid bias in system performance. This is particularly important for systems whose outputs can have a profound impact on people’s lives.
The response may include a description of where the data is collected from and how it is cleaned and verified. For example, methods for handling outliers, missing data and duplicates may be used. Data validation procedures, such as the use of independent test suites or cross-checking, are also important. Automated data quality control tools may be used or data may be audited regularly to ensure that it is up to date.
Detailed documented data quality control methods will help to demonstrate compliance with all regulations. If such processes are not in place, this may indicate potential risks of AI and non-compliance with the requirements of the Regulations.
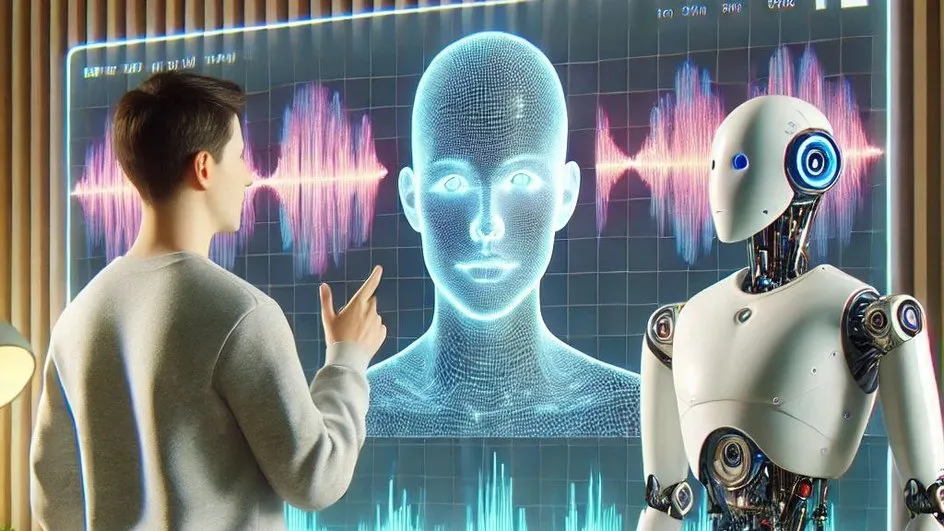
Can human control be realised in the operation of artificial intelligence?
This question is based on Article 14 of the Regulation. It is relevant if your system can be classified as a high risk system. The Regulation requires that humans have the ability to control an AI system in order to minimise risks to the health, safety and fundamental rights of people.
Asking this question is worth considering: realising effective human control over AI systems, especially complex neural networks, leads to serious technical challenges.
Modern deep neural networks often operate as a ‘black box’. Their internal decision-making logic can be extremely complex and incomprehensible to humans. Neural networks can contain millions or even billions of parameters. Its behaviour is often the result of complex non-linear interactions between its components. It is almost impossible to trace the influence of each parameter on the final result without specialised tools. In addition, neural networks can process huge amounts of data at high speed. This makes real-time human monitoring difficult.
Without specialised tools, effective human control over complex AI systems is indeed virtually impossible. Implementing the requirements of Article 14 of the EU AI governance document requires not only organisational measures, but also substantial technical investments in the development and implementation of appropriate tools and methodologies.
What are the possible answers to this question? For example, a system may provide an interface through which an operator can monitor its operation and intervene when necessary. A procedure can be implemented that allows a data subject to stop or cancel the action of the system if something goes wrong.
It is also important to prevent situations where operators rely too much on AI decisions. Mechanisms need to be put in place so that humans can critically evaluate the performance of the system without blindly trusting it.
These measures are necessary to comply with Article 14 of the EU Regulation and ensure the safety and reliability of the AI system. Clear controls increase confidence in the system and reduce the risks of misuse, especially where the system could significantly affect human life.
What documentation is maintained regarding the development and operation of an responsible AI system?
Such documentation is important for transparency so that different stakeholders, from developers to regulators, can understand how the system works and how it was created. In addition, Article 11 obliges the creation and maintenance of up-to-date technical documentation. It is necessary to demonstrate that a high-risk AI system is compliant with regulations. Technical documentation also helps to track the system’s creation and lifecycle. This is important for risk management and auditing.
The documentation may include:
📎 A detailed description of the AI system architecture and its components.
📎 Information about the development processes: methodologies used, tools and milestones.
📎 System design specifications: interfaces and their interaction with the user.
📎 Details of the data used to train and test the AI models.
📎 Description of the machine learning algorithms and methods used in the system.
📎 Data protection impact assessment for the risks associated with AI and the measures taken to minimise them.
📎 Operation logs capturing key events in the operation of the system.
📎 Documentation relating to model testing and validation.
📎 Cybersecurity measures.
📎 Procedures for monitoring system performance and updates.
The documentation should be accessible and understandable to both the testing authorities and the organisations implementing the system. For SMEs, a more simplified form of documentation is acceptable, but it should also be sufficient to fulfil the legal requirements.
How does the system deal with potential biases in the decision-making process?
This is to ensure that AI decisions are fair and do not discriminate against certain groups of people. If the AI makes decisions with biases, it can harm certain groups and lead to legal consequences. Also, unfair decisions may negatively affect its effectiveness and user acceptance. Furthermore, Article 10(2)(f) explicitly obliges AI developers to take measures to combat bias so that their systems comply with the principles of fairness and non-discrimination.
There are several approaches and solutions to combat bias. The first is analysing training data. This allows potential biases to be identified. Through statistical analysis, it is possible to determine how balanced and representative the data is. Model regularisation is another method that helps to reduce model overfitting and reduce the impact of biased data. There are also special algorithms that allow models to consider fairness when training. This helps to eliminate bias in the early stages of development.
Once the system is implemented, it should be continuously monitored to check its performance against biases in real-world scenarios. Explanatory AI tools such as SHAP or LIME can be used for this purpose. They allow us to understand how the system makes decisions and identify hidden biases. Open libraries provide developers with tools to identify and remove biases in machine learning models.
Is user personal information collected during system operation? If so, how are they protected?
This question is asked for several reasons. Firstly, Article 10 requires the implementation of good data management practices for high-risk AI systems in order to comply with legal requirements. Second, proper data governance directly impacts the performance and reliability of AI technologies. Finally, data protection measures will ensure compliance with data privacy legislation as the General Data Protection Regulation (GDPR), which also makes this issue relevant.
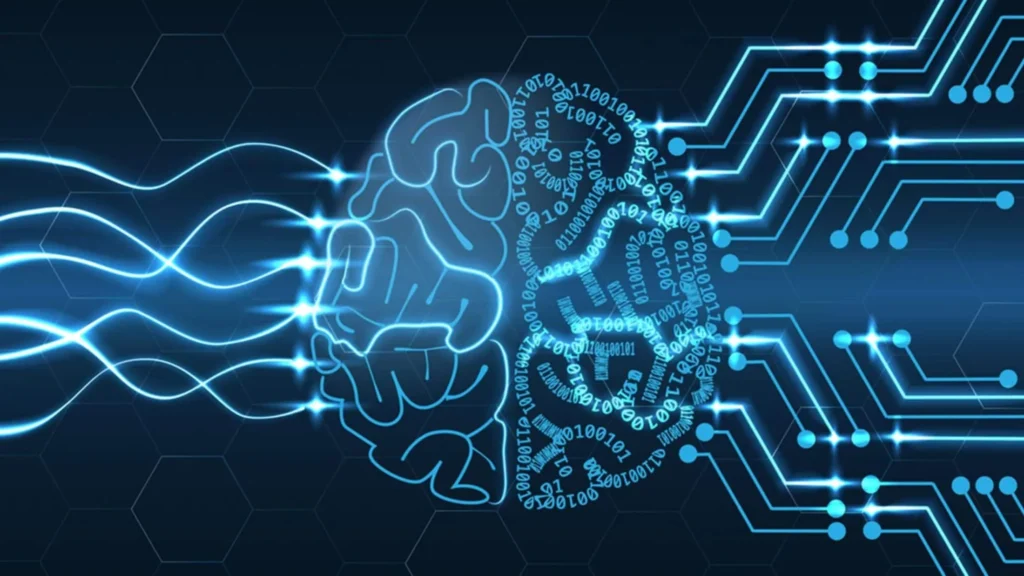
This question can be answered by looking at several key aspects. Firstly, data anonymisation and pseudonymisation techniques should be used to protect the identity of users. Secondly, data should be encrypted both in storage and in transmission to prevent unauthorised access. Also important are data access control policies and user rights management to ensure that only authorised individuals have access to information. Data minimization and data restriction processes help to avoid excessive data collection and the risks associated with data storage. Measures to ensure data integrity and accuracy are also important, as are procedures for regular auditing and monitoring of data processing. It is also important to have mechanisms for deleting or updating outdated data, as well as processes for obtaining and managing users’ consent to the processing of their data. Additionally, you need to protect the system from unauthorised access and data leaks and have clear procedures for handling user requests to access, correct or delete their data.
How does the system handle borderline cases?
Imagine an AI system that is used to recognise faces at the entrance to an office building. Under standard conditions, the system checks employees’ faces against a database and grants access. However, what happens if the employee is partially covered by a scarf, wearing glasses or a hood? If the system is not trained on these scenarios, it can either mistakenly fail to recognise the employee and deny access, or worse, approve access to an unauthorised person. In the event of a failure, this could lead to serious security issues, as well as discrimination if the system is worse at recognising the faces of people with certain appearance features (e.g. dark skin or different facial features).
To comply with the requirements of Article 15, high-risk AI systems must be robust to errors. Also, the system’s ability to handle non-routine situations allows for an assessment of its overall reliability. Proper handling of borderline cases is critical to ensure that AI can be used safely in real-world environments. Effective management of unexpected inputs helps to avoid critical system failures.
The question of how the system handles borderline cases and unexpected inputs may be answered in a variety of ways. Technical experts can describe procedures for testing such cases, including methods for generating extreme and non-standard inputs to ensure that the system can handle them. There may also be a process for validating input data and filtering out incorrect or potentially harmful data.
A variety of methods for testing and handling non-standard situations demonstrate a serious approach to system reliability.The system’s ability to adapt to new types of input data emphasises its flexibility and resilience to changes in the real world.
How does an AI system cope with multi-lingual or cross-cultural inputs?
The ability of an AI system to function effectively in multi-lingual and cross-cultural environments is critical to ensure fairness in the use of AI globally. In an international environment, it is important to accommodate linguistic and cultural differences to avoid discrimination and bias.
Various methods are used to provide multilingual and intercultural capabilities to the AI system. Multilingual natural language processing models that can generate and understand text in multiple languages are used. A variety of datasets are used to train the system, which cover a wide range of cultural expressions, thus minimising bias.
Linguists and cultural experts may even be brought in to work on the system to increase the accuracy of understanding cultural nuances. This helps to account for aspects such as cultural differences in sentiment analysis and semantic parsing. Additionally, mechanisms are implemented to handle multilingual situations where users switch between languages. Speech recognition systems also often take into account different accents and dialects, making interactions with AI more accurate and inclusive.
Either way, having the system able to work in multilingual and intercultural environments demonstrates compliance with the non-discrimination principles enshrined in the EU Regulation.
How is the system updated?
The system must adapt to changes in the environment and to new user requirements. It should be borne in mind that Article 43 of the EU Regulation requires a new conformity assessment in case of significant changes to the system. This is to ensure that the system still meets all necessary standards and requirements. However, in reality, any changes may in fact lead to new risks that need to be carefully assessed and minimised.
The question about upgrade procedures can be answered in a number of ways. Firstly, experts can describe the change management process, including approving and documenting modifications. You can also establish the criteria by which significant changes that require a new compliance assessment are identified. Additionally, you may be provided with details of procedures for testing and validating updates before they are implemented. Mechanisms for monitoring the impact of updates on system performance and security are also important. Having rollback and disaster recovery procedures in place to respond quickly to potential update issues is also important to consider. It is equally important to establish a process for communicating with users about planned and implemented changes, as well as methods for ensuring system continuity during updates.
How does the system handle conflicting or inconsistent data?
Such a question is asked to ensure that the system can handle imperfect data. In real-world environments, data is often incomplete or inconsistent, so the system must be able to deal with it effectively. This is important to maintain its reliability. If the system is unable to properly handle incorrect or inconsistent data, it can lead to inaccurate results and reduced confidence in its performance. In addition, such capabilities of the system increase its resilience to errors. Article 15 of the Regulation directly requires high-risk AI systems to be able to operate in environments where data is incomplete or contains errors.
Possible approaches to address this challenge may include checking data consistency at the data entry stage to ensure that the data is in the correct format and within acceptable limits. The system may also use algorithms to automatically detect anomalies that signal problems with the data. Some systems may apply machine learning techniques to automatically correct or augment data when inconsistencies are detected. Prioritisation systems can be developed to resolve conflicts between data from different sources.
Various methods are used to provide multilingual and intercultural capabilities to an AI system. Multilingual natural language processing models that can generate and understand text in multiple languages are used. A variety of datasets are used to train the system to cover a wide range of cultural expressions, minimising bias. Linguists and cultural experts may even be brought in to work on the system to increase the accuracy of understanding cultural nuances. This helps to account for aspects such as cultural differences in sentiment analysis and semantic parsing. Additionally, mechanisms are implemented to handle multilingual situations where users switch between languages. Speech recognition systems also often take into account different accents and dialects, making interactions with AI more accurate and inclusive.
Either way, having the system able to work in multilingual and intercultural environments demonstrates compliance with the non-discrimination principles enshrined in the EU Regulation.
Data privacy and risks of AI: the conclusion
It is important for lawyers to have a deep understanding of the technical details of how AI systems work in order to effectively assess compliance with regulatory requirements for non-discrimination, security and transparency. Asking the right questions at the right time not only minimises legal risks but also facilitates closer collaboration between legal and technical teams.
Contact us
Fill in the form and we will contact you as soon as possible!